A Quick Technology Update
André Jaun
Chief Technical Officer, Metadvice
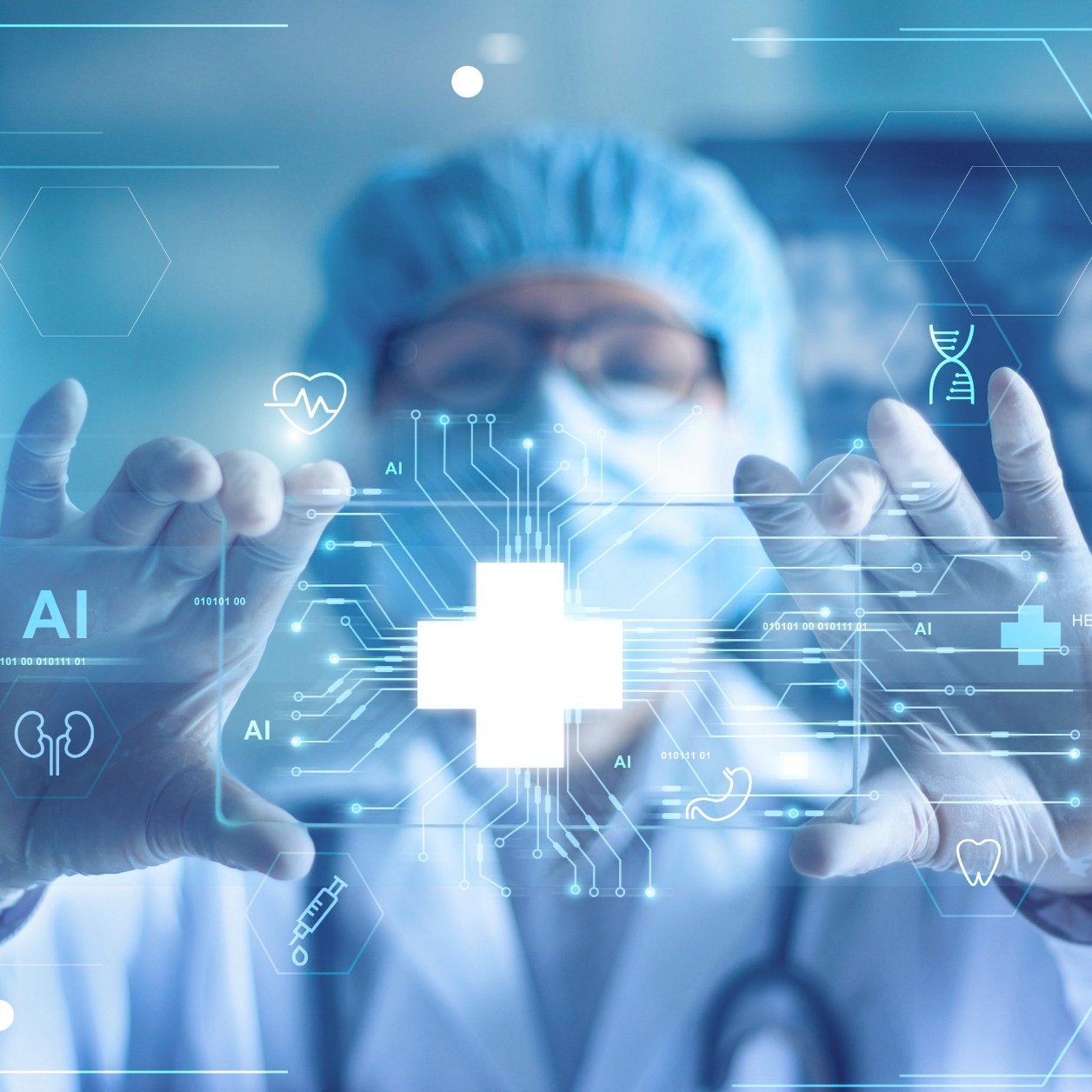
The remarkable progress made with Large Language Models (LLMs) during 2024 warrants a short discussion about how they might be used in Healthcare, barriers that need to be overcome, together with a reality check that bluntly finds GPT-4 inadequate for primary care usage in Sweden.
NOTE This is an opinion piece from the CTO and does not necessarily reflect the view of Metadvice.
What they say.
Because it is easier to discuss what may be feasible rather than to actually implement it, consultants tend to be among the most sanguine when it comes to technology potential. The following reports have been compiled for the year end and concern mainly Generative AI applications:
- WEF – Patient-First Health with Generative AI: Reshaping the Care Experience report. By 2030, the global shortage of healthcare workers is projected to decline from 15 million to 10 million, with generative AI potentially alleviating this gap by augmenting patient triage, disease management, and health education.
- McKinsey & Company – Generative AI in Healthcare: Adoption Trends and What’s Next.
Generative AI solutions will increasingly favor customisation, with 59% of healthcare organizations partnering with vendors and 24% building in-house. - PwC – Essential Eight Technologies for Health Services: AI Leads Investment Priorities.
77% of health executives plan to prioritise AI investment within 12 months to address administrative burdens and enhance patient care. - Forrester – Predictions 2025: Healthcare.
By 2025, generative AI will drive top US insurers’ customer advocacy, and 3 states will enact hospital cybersecurity legislation. - Capgemini – Supercharging the Future of Healthcare with Generative AI.
By 2045, AI could support 50% of healthcare activities, with Gen AI revolutionizing patient care, diagnostics, and drug research. Duke Health – AI Governance in Health Systems: Aligning Innovation, Accountability, and Trust
Health systems must mitigate risks of discrimination from AI tools under new Section 1557 rules for equitable care.IBM – Delivering Responsible AI in Healthcare and Life Sciences
Generative AI will require auditable, explainable outputs and trusted data sources to ensure equitable and trustworthy outcomes in healthcare.
Perhaps because they are also involved with actual deployments, IBM focuses on the requirements that are a prerequisite for a change to the healthcare sector rather than the possibilities that may never lead to a change.
At Metadvice.
Our AI team has been carrying out research with large language models since 2020 when GPT-2 and Bio-BERT where the cutting edge of the field1 and we are maintaining our effort today2 albeit not from a generative modeling point of view. Indeed, Generative AI is only a subset of the LLM capabilities that is particularly well suited for applications with a relatively shallow context.
Multi-modal inputs (combining symptoms, factor measurements, CGM & ECG traces) and real world evidence outcomes (RWE) are likely to be better captured by an extension of one and the same neural network architecture that underpins both the most powerful LLMs and the comorbidity modules that we are developing at Metadvice. In technical terms, the most suitable mathematical representations for reasoning in medicine are vectors and latent spaces and not language.
Because we agree with IBM about a need to provide trustworthy and explainable outputs, we proceed with a limited set of pathologies (e.g. chronic diseases), integrating language (guidelines) and multi-modal data (patient records) in a manner that can be justified (with hyperlinks to the original guideline) and that can also be statistically tested. Yes, we are performing a bespoke clinical trial for each and every individualized recommendation and make sure that similar patients also result in similar outcomes. Apart from opening fruitfull avenues for personalized medicine, this also effectively counters hallucinations that are inherent to neural networks.
A sobering outlook.
While constitutional free speech arguments limit the governments’ authority over patient use3, professional healthcare AI applications are subject to strict regulations. Until clinics can host their LLMs in-house (e.g., using Nvidia Jetson), privacy and security4 concerns will dominate discussions over professional adoption. Moreover, and in spite of its remarkable recent successes,
- Liu et al showing an LLM for disease diagnosis assistance5
current capabilities have been found insufficient for primary care clinical settings in at least two recent papers:
- Bedi et al concluding that Existing evaluations of LLMs mostly focus on accuracy of question answering for medical examinations, without consideration of real patient care data6.
- Arvidson et al concluding that In complex primary care cases, GPT-4 performs worse than human doctors taking the family medicine specialist examination (figure below)7.
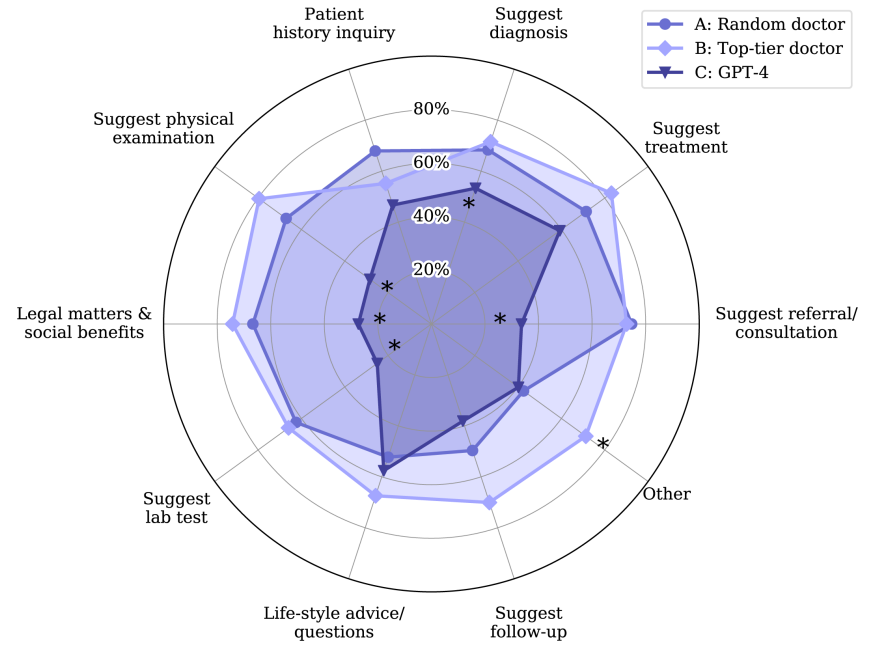
Referring to Arvidson’s figure above, Metadvice observes similar limited adherence from clinicians to clinical guidelines (~50-70% depending on the pathology); rather than falling below performance of routine care (as GPT-4 does), Metadvice models are designed to achieve >95% accuracy against specific guidelines (close to the external circle in the figure).
In addition, our precision medicine (trained with hundreds of thousands of deidentified records that are not accessible to LLMs) is able to improve clinical decision making by taking into account personalized features for each individual and real world evidence outcomes in a comorbid context.
This is why Metadvice continues to deploy tailored neural networks on behalf of our customers in full compliance with regulatory constraints and with a comorbidity angle that can only be captured using a real world evidence perspective — 2025, here we come!
REFERENCES
1 Mathias Minder, Learning Knowledge Graphs from Clinical Guidelines, MSc Thesis, EPFL (2021)
2 Maxime Rufer, Synthetic Clinical Data Generator Using LLMs to Reproduce Medical Guidelines, MSc Thesis, EPFL (2025)
3 Blumenthal et al, Managing Patient Use of Generative Health AI NEJM (2025)
4 Alber et al, Medical large language models are vulnerable to data-poisoning attacks Nat Med (2025)
5 Liu et al, A generalist medical language model for disease diagnosis assistance, Nat Med (2025)
6 Bedi et al. Testing and Evaluation of Health Care Applications of Large Language Models: A Systematic Review, JAMA (2024) Online ahead of print.
7 Arvidson et al, ChatGPT (GPT-4) versus doctors on complex cases of the Swedish family medicine specialist examination: an observational comparative study BMJOpen Vol.14, Issue 12 (2024)