Abstract submitted for the 59th meeting the European Association for the Study of Diabetes,
EASD, Hamburg, October 2–6, 2023
Glucose-Insulin modelling of DM2 patients with CGM monitoring under various therapies
1 UDEM, Inselspital, Freiburgstrasse 2, 3010 Bern, Switzerland
2 Metadvice, Route Cantonale 109, 1025 St-Sulpice, Switzerland
3 Ecole Polytechnique Fédérale de Lausanne, 1015 Ecublens, Switzerland
Background and aims. Continuous glucose monitoring (CGM) provides a wealth of data for diagnostic and therapeutic decision-making in diabetes care. Current clinical treatment guidelines are based on standard time in range statistics. The goal of this study was to build a model, leveraging CGM data only, to characterise glucose-insulin regulation in patients with type 2 diabetes.
Materials and methods. Estimating the rate of oral glucose appearance from the peaks in the CGM response, a non-linear fit of Lotka-Volterra equations is used to estimate insulin-dependent and -independent glucose uptake by tissues, in parallel with the endogenous insulin production. The model was fitted to CGM data from 179 patients with Type 2 Diabetes on various glucose-lowering therapies.
Results. The results suggest that the model adequately captures glucose trajectory in patients with Type 2 Diabetes (as illustrated in Figure 1, below), demonstrating the potential to indirectly quantify biomarkers that are otherwise not directly measurable. These biomarkers may improve the metabolic characterization of patients and personalise the assessment of treatment response beyond a glucose-centric approach.
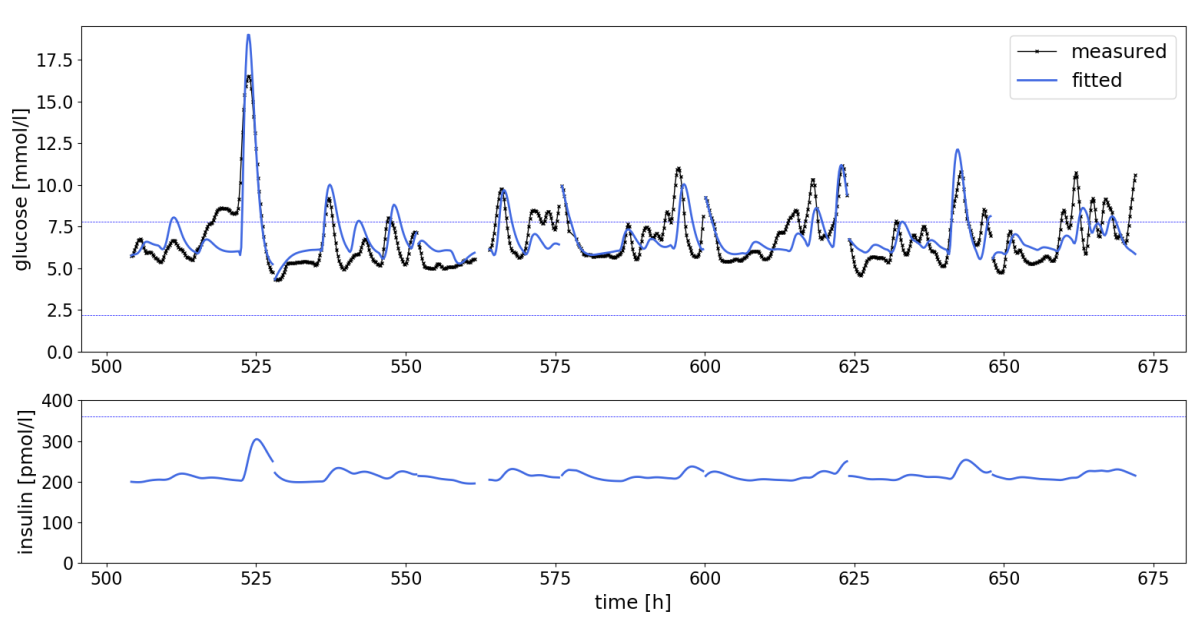
Conclusion. This study presents a novel approach to characterising glucose-insulin regulation in patients with Type 2 Diabetes using CGM data only. The method has the potential to provide valuable insights into hidden biomarkers and improve the assessment of treatment responses, paving the way for more personalised diabetes management strategies. Future work will aim to validate a variety of models in terms of their ability to accurately reproduce physiological parameters in well calibrated clinical settings.
Acknowledgements: Innosuisse – Swiss Innovation Agency (101.082 IP-LS)
Metadvice © 2023